AI for Commodity Price Forecasting: Enhancing Market Predictions with Machine Learning
Client Overview
A major global Phosphate and Potash Mining company faced significant challenges in accurately forecasting commodity prices, which was directly impacting their planning, production, and bottom line.
The Challenge
The client struggled with inaccurate price forecasts for their commodities. This issue was not unique to them, as none of the market leaders in their industry were able to forecast accurately. The inability to predict prices reliably affected their planning and production processes, ultimately hitting their bottom line.
Project Goals
Improve Prediction Accuracy
Improve prediction accuracy for commodity prices
Develop Forecasting Model
Develop an in-house forecasting model
Achieve Accurate Forecasts
Achieve accurate forecasts over horizons of 1, 2, and 3 months
Our Approach: Data-Driven Intelligence
Process Point leverages AI and ML techniques to provide unparalleled insights. Our approach integrates historical data analysis, macroeconomic modeling, market sentiment analysis, and advanced time series models to deliver accurate forecasts and drive operational efficiency.
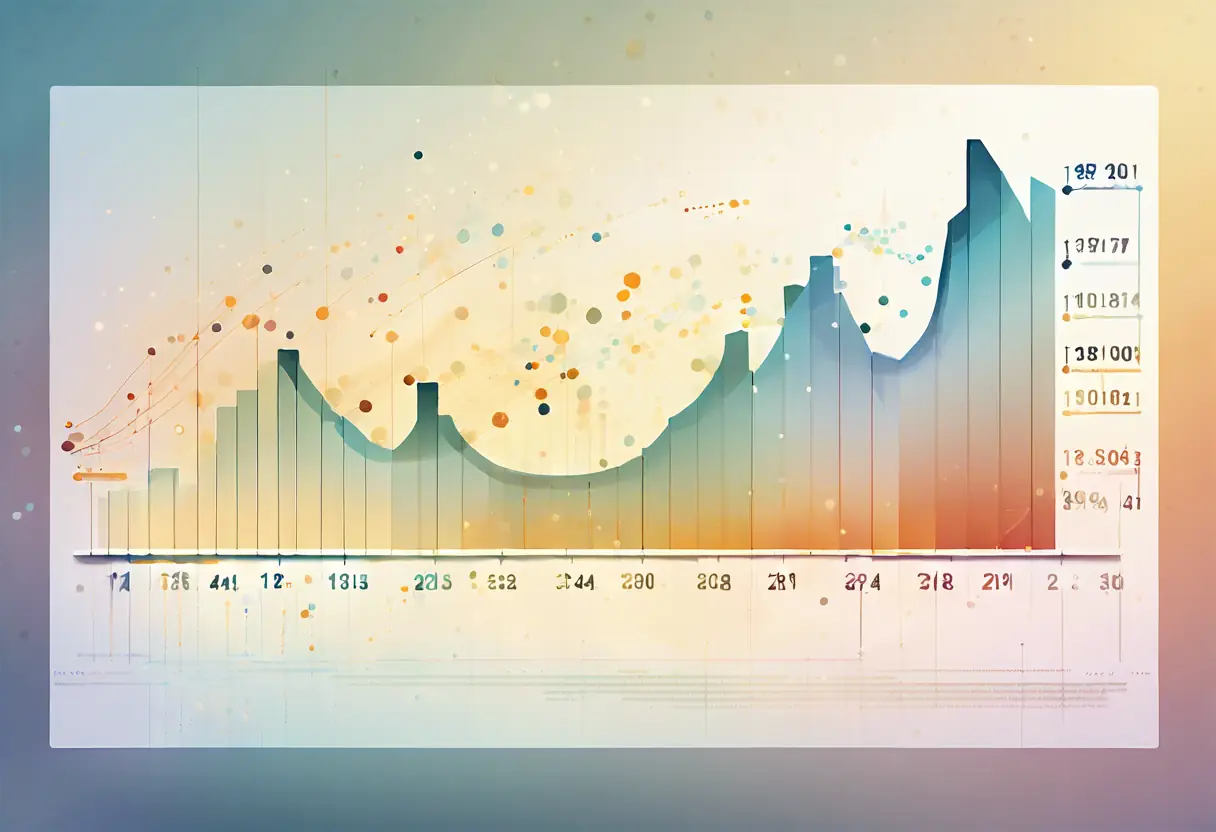
Historical Data Analysis
Collected and analyzed over 200 years of historical data from multiple sources.
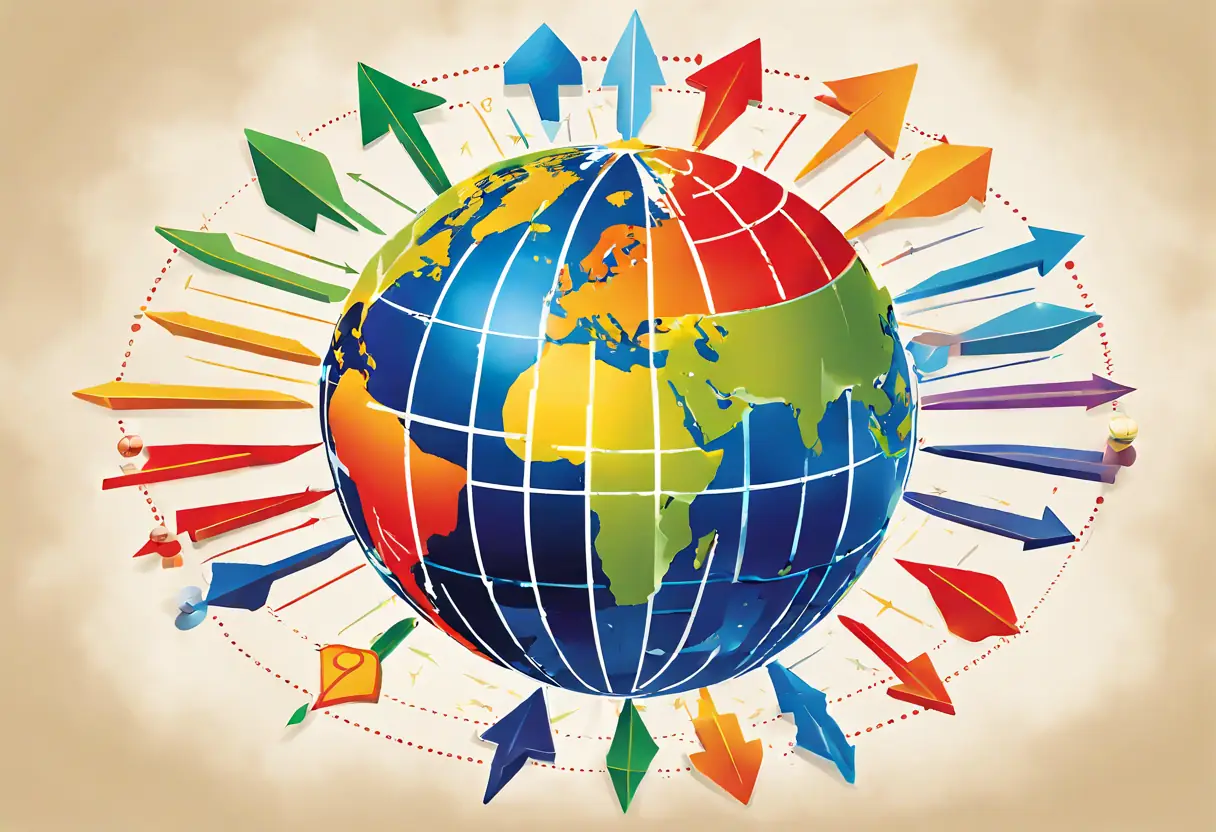
Macroeconomic Modeling
Incorporated macroeconomic factors to capture broader market trends and influences.
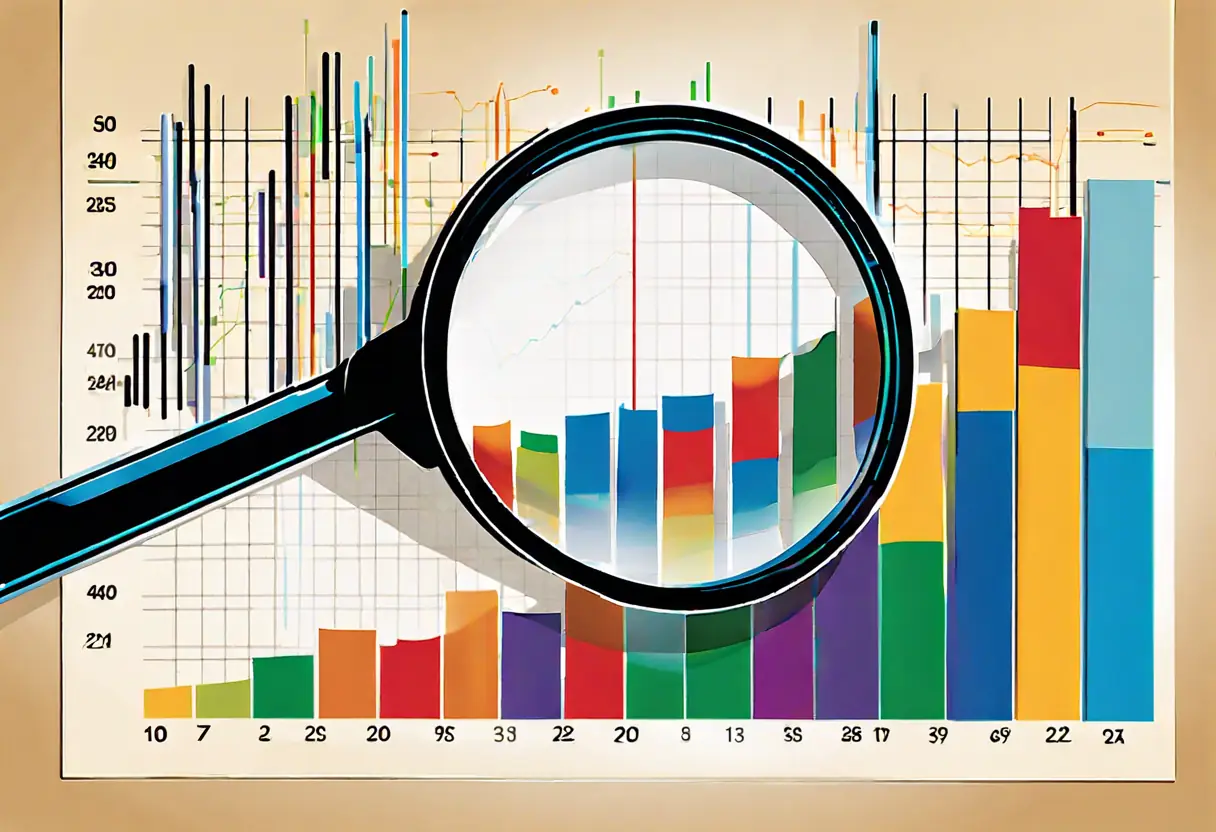
Market Sentiment Analysis
Analyzed market sentiment to gauge short-term price movements and investor behavior.
Results
- Improved Accuracy: Our forecasts showed a significant improvement in accuracy over previous methods and competitor forecasts.
- Better Planning: The client was able to use these more accurate forecasts to improve their planning and production processes.
- Financial Impact: The improved forecasts directly contributed to better decision-making, positively impacting the company's bottom line.
- Customized Solution: By developing an in-house model, the client now has a proprietary tool tailored to their specific needs and market conditions.
Conclusion
- Solution Implemented: Commodity price forecasting tool using advanced data science.
- Approach Taken:
- Application of advanced data science techniques for accurate predictions.
- Comprehensive data analysis to uncover market trends and patterns.
- Key Outcomes:
- Enhanced strategic decision-making for the client.
- Improved forecasting accuracy in the commodities market.
- Data-driven intelligence applied to solve complex business challenges.
- Industry Impact:
- Showcases the power of AI and analytics in commodity trading.
- Demonstrates the value of predictive insights in volatile markets.